Video Quality Metrics
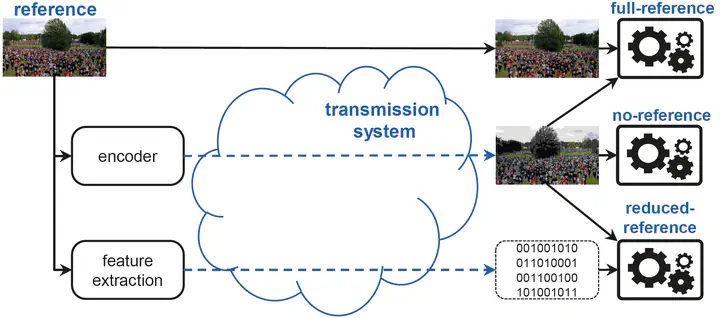
Video quality metrics provide models that allow as us to predict the subjective visual quality from objectively measurable properties of the video sequences under test utilising a set of different features in a prediction model to provide an estimation of the video quality. Typical features are blur or blockiness, describing how strongly details have been lost e.g. due to the loss of high frequency components in quantisation and how visible blocks are e.g. due to block-based transformations in video coding, respectively.
One aim of video quality metrics is obviously to deliver quality predictions that are equivalent to the results from subjective testing, but additionally one major goal is also that the prediction can be performed in all situations. Therefore the focus of my contributions is on so-called no-reference video quality metrics that allow the video quality prediction without the availability of an undistorted reference.
Key publications: